PCA in the NBA
Note this post was written before the 2019-20 season began, while I was still figuring out how to set up my website. Some of the writing reflects this fact, particularly the Team Ramblings section. Look for my update at the All Star Break where I'll post about how the 2019-20 season shapes up in terms of PCA.
What these youngbloods have to understand
is that this game has always been, and will always be, about buckets.
-Bill Russell
Throughout the 2018-19 regular season NBA teams took, on average, about 89 shots per game, that's less than 23 a quarter. In a game all about buckets, there really aren't many to go around. With shots being such a limited resource, their allocation can (and already has) greatly alter(ed) the game as we know it.
Ever since the advent of Moreyball there's been a steady flow of takes on the shift towards the three heavy offense. Now, the point of this post is not to be the Kent Brockman of threes - I for one welcome our new three point overlords! - nor wax poetic about behemoths methodically backing each other down in the post. Rather, this post will introduce a novel way of examining the distribution of a team's shots around the court. In particular, a very popular data science technique, principal components analysis (PCA), will be applied to raw shot distribution data.
The Data
The NBA began accurately tracking the x,y position of every shot taken during a game back in the 2000-01 regular season. Using the python package nba_api this data can be collected and analyzed. Every single post up, fade-away jumper, or step-back three taken occurs in one of 15 sections of a court, outlined below.
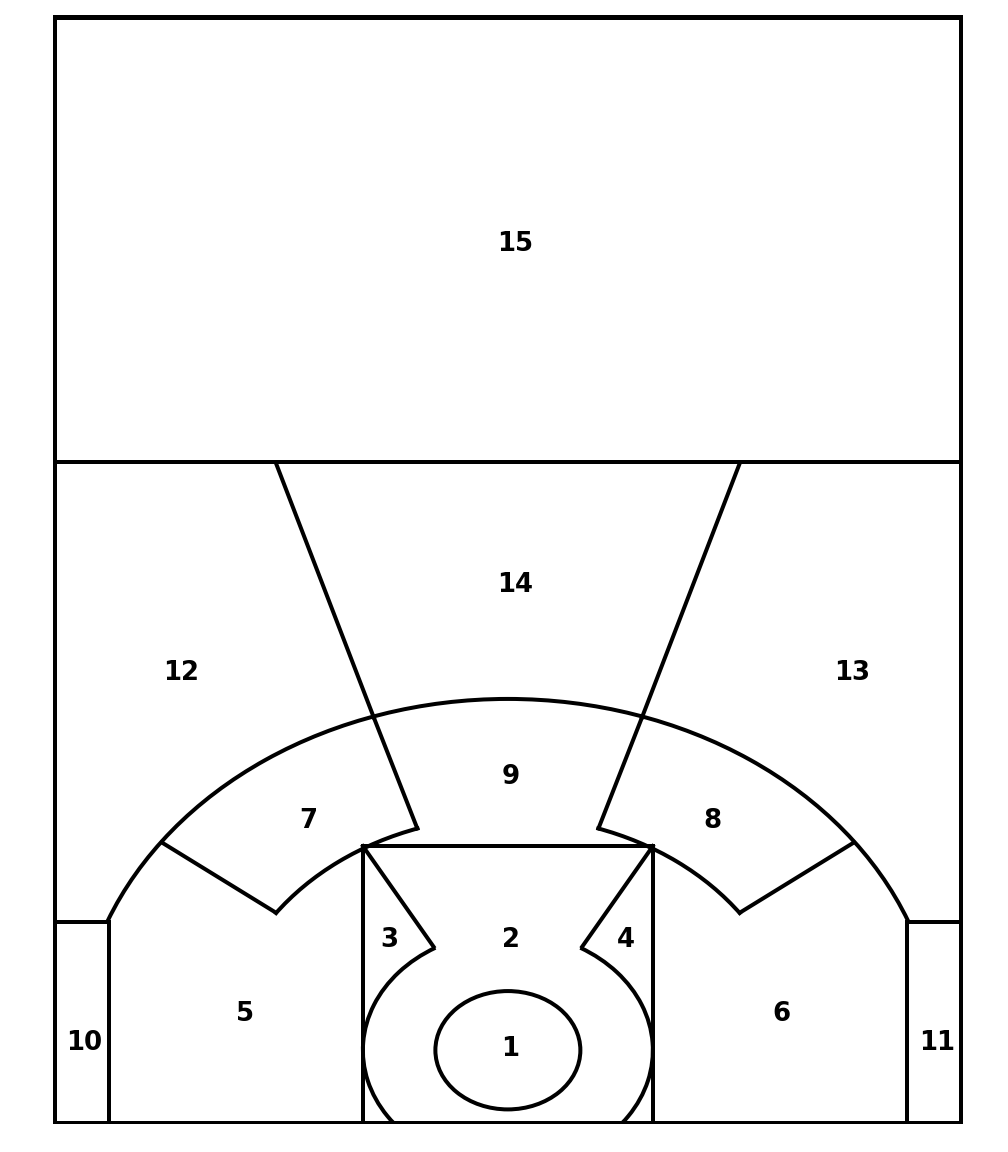
ID | Zone Name |
---|---|
1 | Restricted Area |
2 | In the Paint, Center |
3 | In the Paint, Left |
4 | In the Paint, Right |
5 | Mid-Range, Left |
6 | Mid-Range, Right |
7 | Mid-Range, Center-Left |
8 | Mid-Range, Center-Right |
9 | Mid-Range, Center |
10 | Left Corner 3 |
11 | Right Corner 3 |
12 | Above the Break 3, Left |
13 | Above the Break 3, Right |
14 | Above the Break 3, Center |
15 | Backcourt |
With the shot data from the NBA's official stats, each atttempt can be classified as occurring in one of these 15 zones. A team's shot zone distribution can then be created by taking the percentage of a team's total attempts that occurred in each zone. For example, here is the shot distribution for the 2018-19 World Champion Toronto Raptors.
Zone | % of Toronto's Attempts |
---|---|
Restricted Area | 32.16 |
In the Paint, Center | 14.31 |
In the Paint, Left | 0.55 |
In the Paint, Right | 0.68 |
Mid-Range, Left | 3.38 |
Mid-Range, Right | 3.75 |
Mid-Range, Center-Left | 1.74 |
Mid-Range, Center-Right | 2.53 |
Mid-Range, Center | 3.01 |
Left Corner 3 | 5.52 |
Right Corner 3 | 5.31 |
Above the Break 3, Left | 9.16 |
Above the Break 3, Right | 9.71 |
Above the Break 3, Center | 7.98 |
Backcourt | 0.22 |
These shot distributions provide a snapshot of how a team allocated its limited resource in a particular season. This is great, however, it is slightly unmanageable to track how 15 numbers change over the course of 20 or so seasons. It would be nice if we could reduce that to one or two numbers to track over time.
PCA All The Way
Enter Principal Components Analysis (PCA). PCA takes high dimensional data sets and reduces them to a more manageable number of dimensions. For example, PCA can take these 15 percentages and boil them down to just 1 or 2 numbers (Click to go to a link explaining PCA). Running the 2018-19 Toronto Raptors' shot distribution through PCA produces the following two values: (0.093,-0.030). Doing this for every team's shot distribution from the 2000-01 to the 2018-19 seaons produces the following plot, where a team's first PCA value is on the horizontal axis, and the second on the vertical.
Season:
Team:
Shots in the Paint:
Shots from Midrange:
Shots from 3:
Run, don't walk from the Blob of data points! Don't worry this blob can make sense. When data is put through PCA, something called principal component vectors are also produced. These explain what the values mean in terms of the original data set. Here are the first two component vectors:
Zone | First Principal Component Score |
---|---|
Mid-Range, Left | -0.581 |
Mid-Range, Right | -0.483 |
Mid-Range, Center-Right | -0.159 |
Mid-Range, Center-Left | -0.150 |
Mid-Range, Center | -0.055 |
In the Paint, Left | -0.020 |
In the Paint, Right | -0.012 |
Backcourt | 0.002 |
In the Paint, Center | 0.057 |
Left Corner 3 | 0.111 |
Right Corner 3 | 0.113 |
Above the Break 3, Center | 0.259 |
Restricted Area | 0.297 |
Above the Break 3, Right | 0.307 |
Above the Break 3, Left | 0.314 |
Zone | Second Principal Component Score |
---|---|
Above the Break 3, Left | -0.243 |
Above the Break 3, Right | -0.232 |
Above the Break 3, Center | -0.208 |
In the Paint, Center | -0.138 |
Left Corner 3 | -0.092 |
Right Corner 3 | -0.073 |
In the Paint, Left | -0.015 |
In the Paint, Right | -0.013 |
Mid-Range, Center | -0.010 |
Backcourt | 0.001 |
Mid-Range, Left | 0.010 |
Mid-Range, Center-Left | 0.024 |
Mid-Range, Center-Right | 0.044 |
Mid-Range, Right | 0.048 |
Restricted Area | 0.897 |
What do these two vectors mean? For any principal component vector, positive (meaning greater than 0) vector entries correspond to more positive principal component values. For example a team with 100% of their shots from Above the Break 3, Left will have as positive a first principal component value as possible because that is the most positive row of the first principal component vector table. On the other hand, a team that shot 100% of their shots from Mid-Range Left will have as negative (less than 0) a first principal component value as possible because that is the most negative row of the first principal component vector table.
When it comes to the plot above, this means that teams with a larger percentage of their shots from the mid-range will be on the left (the negative side) of the chart, while teams shooting more from deep and in the restricted area will be on the right side (the positive side).
This particular grouping of court regions has been a hot topic in the discussion of shot efficiency. In recent years it has been discovered that mid-range shots are "less efficient" than their close and long-range counterparts. Thus the first principal component value is negative for those "less efficient" teams that shoot more from mid-range, while the first principal component value is positive for "more efficient" teams that tend to shoot from three and under the rim. In terms of the earlier plot, more efficient teams step to the right, less efficient teams to the left please.
Coloring each dot by the team's percentage of total shots taken from three or the restricted area paints this picture. Going from left to right on the PCA plot below increases the percentage of total attempts from downtown or under the basket. A team's first PCA value captures the "efficiency" of their shot selection.
The second PCA value captures whether a team prefers to shoot threes or close-up twos from the restricted area. Three point zones are the most negative in the second principal component vector, while the restricted area is overwhelmingly the most positive. Teams that live under the rim will be found near the top of the PCA value plot, while teams shooting it from deep in the [approximate name of arena where game is being played] can be found near the bottom. Check it out in the following two plots.
These PCA scores have value in tracking the how a team allocates its shot attempts around the court. The first PCA value can be thought of as a shot distribution Efficiency Measure, with a positive value indicating an "efficient" team, and a negative value an "unefficient" team. The second PCA value can then be considered a way to measure a team's tendency to shoot from three or close range, with negative values meaning more threes and positive values meaning more shots at the basket.
Crawling Towards Efficiency
With the success of the Houston Rockets' three heavy offense, much has been made of the NBA's transition into a gun slinger league. Mathematically this transition does make sense. According to research performed by NBA analyst and geographer Kirk Goldsberry, the restricted area and just beyond the arc are the only spots on the court with a positive return on investment, meaning the only places where the expected points per shot is above 1. It is pretty straight forward, 23 shots from spots with expected points per shot above 1 should net more than 23 points, 23 shots from spots with expected points per shot below 1 should net less than 23 points. As a reminder, the team with more points typically wins the game.
It's a copycat league, so as Houston started to have success against the game's Goliath State Warriors, other teams started to follow suit devising shot distributions that primarily feature these "efficient" zones of the court. If this is true, it should be reflected in the PCA chart. Click the 'Start!' button of the graphic below to see how PCA values have progressed over the last 20 seasons.
And there it is. As the seasons progress there is a clear shift from the left of the chart (the area where less efficient midrange heavy teams live), to the right of the chart (where the threes and restricted areas roam), as expected.
Team Ramblings
Beyond the NBA wide season to season transitions these PCA coordinates can be used to examine the shot distributions of particular teams. What follows are four vignettes on individual team PCA transitions.
If it Ain't Broke Don't Fix it
The Warriors have the most prolific shooting duo in history, Steph and Klay, so it's a no brainer that they take advantage of this more efficient approach, right? Well yes, but actually no. Click the 'Start!' button on the below plot to watch Golden State's progression since 2014 compared to the rest of the NBA.
One refrain throughout the 2018-19 season explaining the Warriors struggles (if a fifth straight finals birth can be called that) was that the rest of the NBA got better. Did it, or did it just get smarter?
The Warriors started their dynasty run near the front of the herd, when it came to shot distribution. In their record breaking 2015-16 season they placed their shots more "efficiently" than all but two teams in the league, with over 35% of their shots coming from three (second to only the Houston Rockets). Then they got stagnant. After the 2015-16 season they watched the rest of the association pass them by, GSW ended the 2018-19 season as the team with the second highest percent of shots from mid-range (second only to the three detesting Spurs).
But the Warriors went 3 for 5 in the NBA finals, and probably would have gone 4 for 5 if either of Klay or KD don't get hurt (sorry Raptors fans, but its true). So does shot distribution relative to the rest of the league even matter? If a team features four future hall of famers, probably not so much, especially when 2 (possibly 3) are hall of famers because of their ability to make efficient shots. Klay and Curry are so good at converting threes that their team can get away without taking as many as the rest of the NBA.
For the second half of this decade the Warriors more or less ran back the same shot distribution season after season, to historic success. If it ain't broke don't fix it. The thing is, it might actually be broke. Heading into the 2019-20 season the Warriors will have to replace Kevin Durant and Klay Thompson (temporarily at least) with D'Angelo Russell. While D'Angelo is a rising star, it is insanity to think Steve Kerr could just slot him into the same offensive scheme and expect similar results. It seems likely they will make a shift toward the right of the PCA chart.
Houston We Have a Harden
No team is more renowned (or even reviled) for their reliance on threes and layups than the Houston Rockets. While these more "efficent" shot distributions have been deemed "Moreyball" the PCA plot reveals that it was not Daryl that led this transition. Morey has been GM of the Rockets since 2007, but Houston did not significantly change its shot distribution until a few years later. Click the below plot to see what team events do coincide with noticeable changes in shot distribution.
Maybe a better name would be "Hardenball"? Now it could be argued that Daryl discovered the competitive advantage that 3 > 2, traded for a player that is perfect for such an offense, and then hired a coach that would take that offense to the extreme. But, it is equally likely that Morey just traded for a good player that was (stupidly) available, and then built an offensive system around said player that would help him, and the team, succeed. Either way, it's clear that it was not what we call Moreyball until James Harden touched down in Houston.
The Process Was About More Than Tanking
In 2013 NBA analytics martyr Sam Hinkie (and current head coach Brett Brown) was hired by the 76ers to begin their process. While the "The Process" has become synonymous with years of losing to accrue future assets, it also signified a major shift in the Sixers' shot allocation. Again, click the plot below to observe.
In that inaugural process season, Philadelphia had one of the most "efficient" shot distributions in the whole NBA. Not only that, their jump from 2012-13 to 2013-14 represents one of the largest season to season shifts on the PCA plot. However, similar to the Warriors, they stopped moving forward. This could be due to their consistent personnel decisions, opting to draft players that can't shoot, or it could be the one constant the team has shared every season since The Process began, Coach Brett Brown.
It will be interesting to see how the Sixers' shot distribution develops this upcoming season. They lost their best gunner to the Pelicans this offseason, but Simmons has allegedly been working on his jumper all Summer. The 2019-20 season could provide further evidence that shot distribution is a characteristic of the coach, if it stays in the familiar Philadelphia spot.
LeBron Who?
When LeBron declared he was 'Coming Home' in the summer of 2014, the Cleveland Cavaliers became the perennial Eastern Conference representative in the NBA finals. The return of the King did not merely alter the title odds for his home town team, it also signified a shift in their shot distribution.
However, when LBJ left for purpler pastures he did not take the Cavaliers' shot distribution with him. Click the plot below.
Despite losing the star of Space Jam 2, Cleveland placed its attempts in a near identical manner. This seems somewhat odd, since much of the Cavaliers' offense was predicated on LeBron, particularly in the 2017-18 run. It can't really be chalked up to roster consistency, the Cavaliers roster was somewhat of a revolving door in the 2018-19 season as the team attempted to address a deluge of injuries as well as clean up their cap situation moving forward. It could be argued that this is a remnant of the coaching staff that was in place during LeBron's second stint, but the Cavaliers notably lost head coach Tyronn Lue. Perhaps interim coach Larry Drew was the offensive mastermind this entire time.
Whatever the reason, it wouldn't be surprising to see a massive departure from their current PCA cluster this coming season. With an entirely new coaching staff, and an influx of freshly drafted talent, the Cavaliers will probably see change in 2019.
Kenny Atkinson Was a Nets Gain
Three years after one of the worst trades in NBA history, the Nets brought in Kenny Atkinson to help right the ship. While it took Atkinson three seasons at the helm to bring a season with a winning record (42-40, so just barely), what he immediately brought was a new shot distribution strategy. Click the plot below.
Throughout Atkinson's tenure the Nets have been one of the furthest right teams on the PCA chart. Typically they trailed only Houston, and in 2018-19 they were one of four teams to shoot over 90% of their shots from outside of midrange. Atkinson has leaned into Moreyball hard, but not to much success. This coming season will be interesting as it marks Atkinson's first season with etablished all stars. How will he integrate Kyrie Irving and Deandre Jordan into this system?
What PCA Has to Say
These PCA coordinates have allowed an examination of the macro shot distribution trends of the NBA as well as the micro trends that season to season team decisions produce. The two numbers provide a novel way of analyzing team shot distributions beyond the three, mid-range, and paint shot percentages. While those percentages will always be important, the procedure presented above is a nice supplement to the standard analyses.
For one, when the shot distribution percentages are run through the PCA algorithm there is no prior knowledge or bias that suggests the importance of the three point line before the algorithm is run. PCA picked up on this trend ignorant of basketball talking heads. PCA allows the data to speak for itself.
This feature will be important going forward. The 2018-19 season highlighted how much teams are finally buying into the efficiency of their shot distributions. Much like on-base percentage in baseball, the fact that 3 > 2 is no longer a competive advantage. In hindsight the shift from long twos to threes was obvious, but when the three point line was introduced nobody took it seriously. People will likely not see the next innovation coming, if they did teams would already be doing it. PCA could help identify the next trend as its happening rather than the six or so years it took for everyone to catch up with Houston. PCA could be a canary in the shot distribution coal mine.